Mingren Shen
I am a software developeer and machine learning engineer now.
I graduated from UW-Madison with Ph.D. and M.S. in Material Sciences and M.S. in Computer Sciences, where I work on applying computer vision and machine learning methods into material and related area problems.
Before UW-Madison, I got B.S. Physics at Nanjing University and M.S. Physics at University of Chinese Academy of Sciences where I was advised by Dr. Ke Chen and Dr. Mingcheng Yang .
I obtained M.S. in Computer Sciences at UW-Madison in May 2019. Cheers!
I obtained M.S. in Material Sciences and Engineering at UW-Madison in December 2020. Cheers!
I obtained Ph.D. in Material Sciences and Engineering at UW-Madison on December 26th, 2021. Cheers!
Email  / 
CV  / 
Google Scholar  / 
LinkedIn
|
Work Experience
I'm interested in computer vision, machine learning, image processing, virtual reality, and computational material and biology. Much of my research is about applying newly developed computational methods and models for the physical world including DNA, TEM images etc.
|
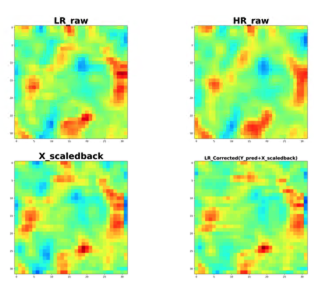 |
Computing High-Fidelity PDE Solutions on Coarse Meshes using Machine Learning
Minren Shen, Summer 2021 Internship
Advisor: Rishikesh Ranade, Jay Pathak, Ansys Inc
Computational fluid dynamics simulations are important tools for studying of fluid flow involved phenomena, such as climate changes, weather systems, nuclear fusion plasma, vehicle aerodynamics structure designs etc. All those processes can be described by Naiver-Stokes Equation but to solve Naiver-Stokes Equation in fine scale requires huge amounts of computation resources. Here we propose a machine learning strategy to calculate high fidelity solutions on coarse meshes. Our approach uses a convolutional neural network to correct the lower resolution 3D flow fields computed by PDE solvers to higher resolution ones with adding of small-scale features that are not contained in lower resolution simulations. The correction is predicted on each mesh element and is a function of the solution fields and their gradients on that element as well as its neighbors. Initial results indicate the neural network can accelerate the CFD simulations with fewer resources and generate flow field that is comparable to higher resolution simulations. This study shows that applying machine learning tools could pave the way for faster CFD simulations.
|
Publications Lists and Abstract
|
-
Mingren Shen, Dina Sheyfer, Troy David Loeffler, Subramanian K.R.S. Sankaranarayanan, G. Brian Stephenson, Maria K.Y. Chan and Dane Morgan. "Machine learning for interpreting coherent X-ray speckle patterns." Computational Materials Science 230 (2023): 112500.
-
Jacobs, Ryan, Mingren Shen, Yuhan Liu, Wei Hao, Xiaoshan Li, Ruoyu He, Jacob RC Greaves et al. "Performance and limitations of deep learning semantic segmentation of multiple defects in transmission electron micrographs." Cell Reports Physical Science (2022): 100876.
-
Awe, Adam M., Michael M. Vanden Heuvel, Tianyuan Yuan, Victoria R. Rendell, Mingren Shen, Agrima Kampani, Shanchao Liang, Dane D. Morgan, Emily R. Winslow, and Meghan G. Lubner. "Machine learning principles applied to CT radiomics to predict mucinous pancreatic cysts." Abdominal Radiology (2021): 1-11.
-
Mingren Shen, Guanzhao Li, Dongxia Wu, Yuhan Liu, Jacob Greaves, Wei Hao, Nathaniel J. Krakauer, Leah Krudy, Jacob Perez, Varun Sreenivasan, Bryan Sanchez, Oigimer Torres, Wei Li, Kevin Field, and Dane Morgan. "Multi defect detection and analysis of electron microscopy images with deep learning." Computational Materials Science 199 (2021): 110576.
-
Mingren Shen, Guanzhao Li, Dongxia Wu, Yudai Yaguchi, Jack C. Haley, Kevin G. Field, and Dane Morgan. "A deep learning based automatic defect analysis framework for In-situ TEM ion irradiations." Computational Materials Science 197 (2021): 110560.
-
Kevin G. Field, Ryan Jacobs, Mingren Shen, Matthew Lynch, Priyam Patki, Christopher Field, and Dane Morgan. "Development and Deployment of Automated Machine Learning Detection in Electron Microcopy Experiments." Microscopy and Microanalysis 27, no. S1 (2021): 2136-2137.
-
Gurbani, Sidharth, Dane Morgan, Varun Jog, Leo Dreyfuss, Mingren Shen, Arighno Das, E. Jason Abel, and Meghan G. Lubner. "Evaluation of radiomics and machine learning in identification of aggressive tumor features in renal cell carcinoma (RCC)." Abdominal Radiology (2021): 1-11.
-
Field, Kevin G., Mingren Shen, Caleb P. Massey, Kenneth C. Littrell, and Dane D. Morgan. "Rapid Characterization Methods for Accelerated Innovation for Nuclear Fuel Cladding." Microscopy and Microanalysis 26, no. S2 (2020): 868-869.
-
Xiaoyu Sun, Nathaniel J. Krakauer, Alexander Politowicz, Wei‐Ting Chen, Qiying Li, Zuoyi Li, Xianjia Shao, Alfred Sunaryo, Mingren Shen, James Wang, Dane Morgan. Assessing Graph‐based Deep Learning Models for Predicting Flash Point. Molecular Informatics (2020), 39 , 1900101.
-
Mingren Shen, Rui Liu, Ke Chen, and Mingcheng Yang. "Diffusive-Flux-Driven Microturbines by Fore-and-Aft Asymmetric Phoresis." Physical Review Applied 12, no. 3 (2019): 034051.
-
Yilin, Liu, Gregory R. Kirk, Brendon M. Nacewicz, Martin A. Styner, Mingren Shen, Dong Nie, Nagesh Adluru, Benjamin Yeske, Peter A. Ferrazzano, and Andrew L. Alexander. Harmonization and Targeted Feature Dropout for Generalized Segmentation: Application to Multi-site Traumatic Brain Injury Images. In Domain Adaptation and Representation Transfer and Medical Image Learning with Less Labels and Imperfect Data, pp. 81-89. Springer, Cham, 2019.
-
Luo, Guan-Zheng, Ziyang Hao, Liangzhi Luo, Mingren Shen, Daniela Sparvoli, Yuqing Zheng, Zijie Zhang et al. N 6-methyldeoxyadenosine directs nucleosome positioning in Tetrahymena DNA. Genome biology 19, no. 1 (2018): 200.
-
Mingren Shen, Fangfu Ye, Rui Liu, Ke Chen, Mingcheng Yang, and Marisol Ripoll. Chemically driven fluid transport in long microchannels. The Journal of chemical physics 145, no. 12 (2016): 124119
-
Mingren Shen, Liu Rui, Hou Mei-Ying, Yang Ming-Cheng, and Chen Ke. Mesoscale simulation of self-diffusiophoretic microrotor. ACTA PHYSICA SINICA 65, no.17 (2016).
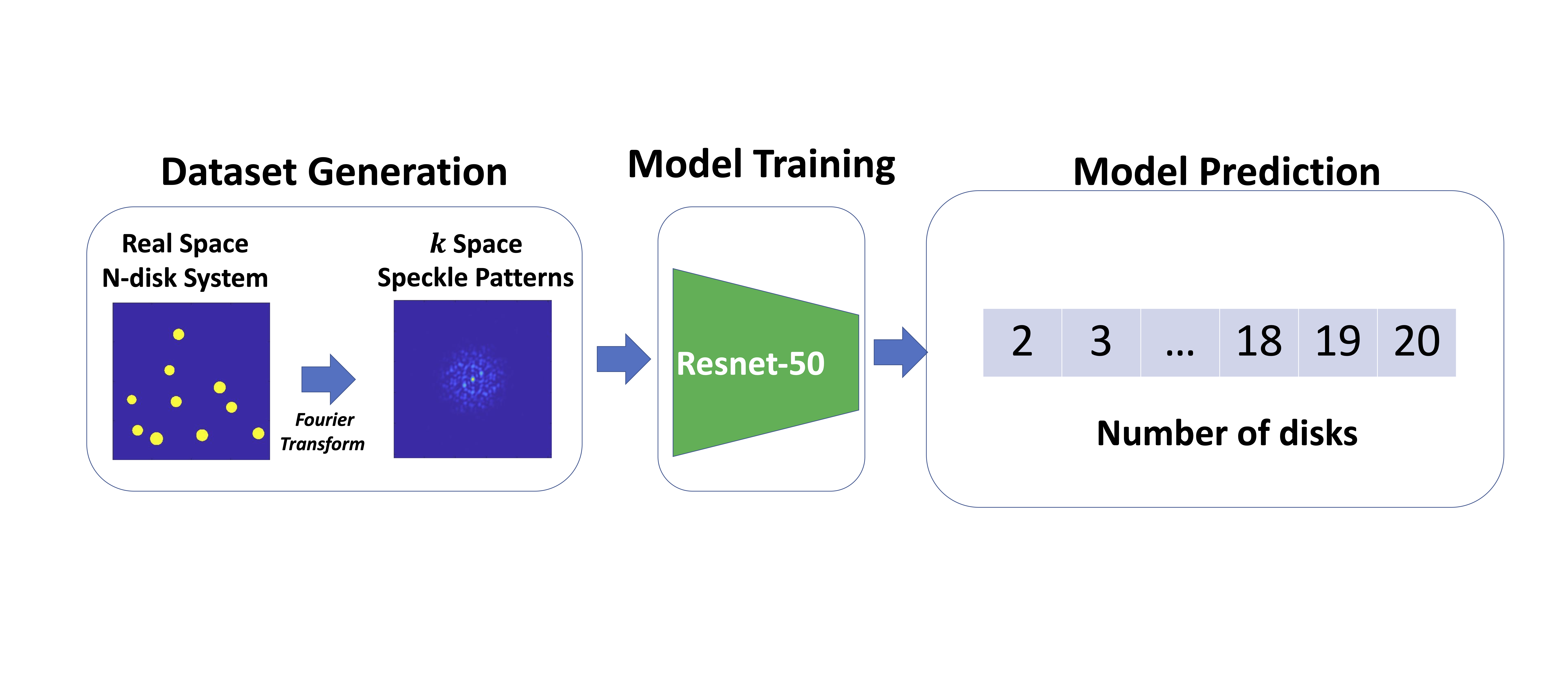 |
Machine learning for interpreting coherent X-ray speckle patterns
Mingren Shen, Dina Sheyfer, Troy David Loeffler, Subramanian K.R.S. Sankaranarayanan, G. Brian Stephenson, Maria K.Y. Chan and Dane Morgan. 2023
Speckle patterns produced by coherent X-ray have a close relationship with the internal structure of materials but quantitative inversion of the relationship to determine structure from speckle patterns is challenging. Here, we investigate the link between coherent X-ray speckle patterns and sample structures using a model 2D disk system and explore the ability of machine learning to learn aspects of the relationship. Specifically, we train a deep neural network to classify the coherent X-ray speckle patterns according to the disk number density in the corresponding structure. It is demonstrated that the classification system is accurate for both non-disperse and disperse size distributions.
Paper Link
|
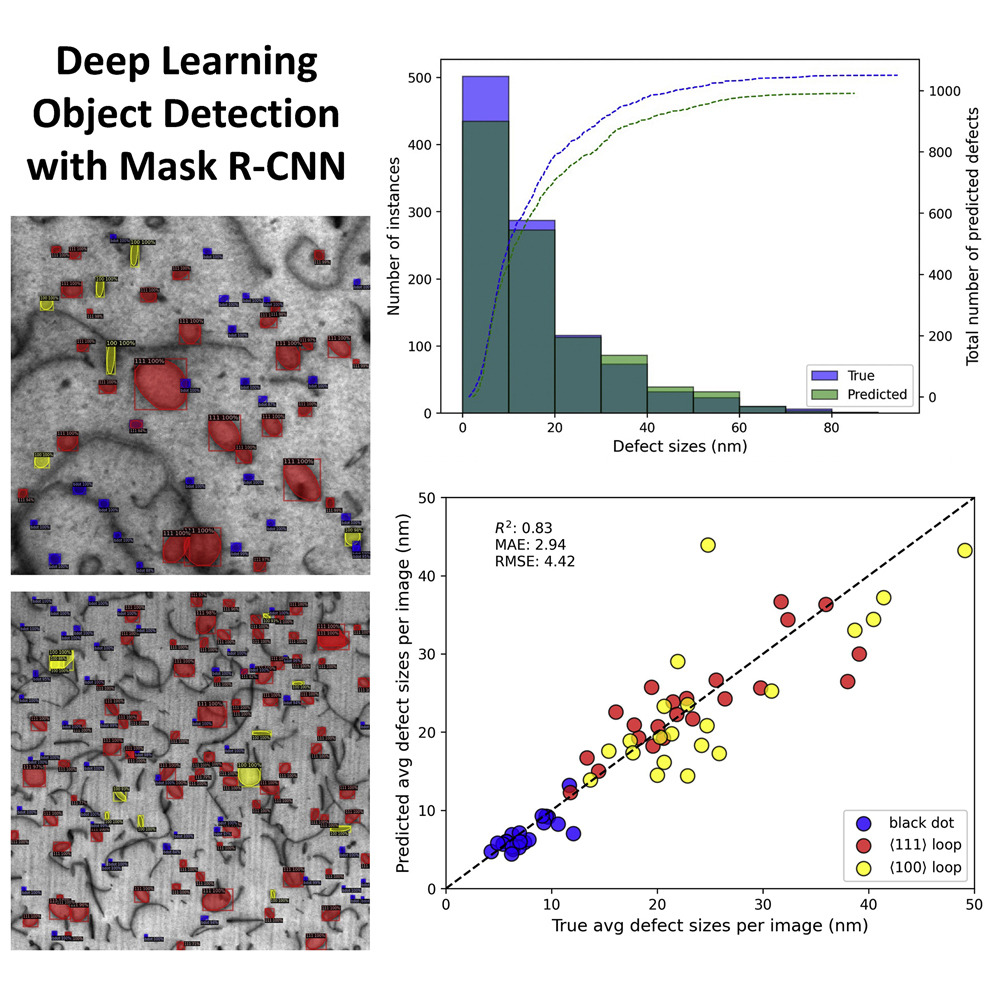 |
Performance and limitations of deep learning semantic segmentation of multiple defects in transmission electron micrographs
Ryan Jacobs, Mingren Shen, Yuhan Liu, Wei Hao, Xiaoshan Li, Ruoyu He, Jacob RC Greaves, Donglin Wang, Zeming Xie, Zitong Huang, Chao Wang, Kevin G Field, Dane Morgan. 2022
Transmission electron microscopy (TEM) is a popular method for characterizing and quantifying defects in materials. Analyzing digitized TEM images is typically done manually, which is a time-consuming and potentially error-prone task that is not scalable to large dataset sizes, motivating development of automated methods for quantifying and analyzing defects in TEM images. In this work, we perform semantic segmentation of multiple defect types in electron microscopy images of irradiated FeCrAl alloys using a deep learning mask regional convolutional neural network (Mask R-CNN) model. We evaluate the performance of the model based on distributions of defect shapes, sizes, and areal densities relevant to informing physical modeling and understanding irradiated Fe-based materials properties. To better understand the performance and present limitations of the model, we provide examples of useful evaluation tests, which include a suite of random splits and dataset-size-dependent and domain-targeted cross-validation tests, exposing potential weak points in the model applicability domain. Our model predicts the expected irradiation-induced material hardening to within 10–20 MPa (about 10% of total hardening), on par with experimental error. Finally, we discuss the first phase of an effort to provide an easy-to-use, open-source object detection tool to the broader community for identifying defects in new images.
Paper Link
|
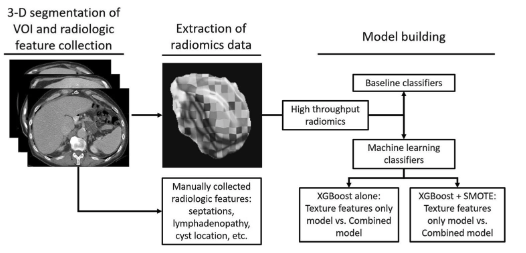 |
Machine learning principles applied to CT radiomics to predict mucinous pancreatic cysts
Awe, Adam M., Michael M. Vanden Heuvel, Tianyuan Yuan, Victoria R. Rendell, Mingren Shen, Agrima Kampani, Shanchao Liang, Dane D. Morgan, Emily R. Winslow, and Meghan G. Lubner. 2021
Current diagnostic and treatment modalities for pancreatic cysts (PCs) are invasive and are associated with patient morbidity. The purpose of this study is to develop and evaluate machine learning algorithms to delineate mucinous from non-mucinous PCs using non-invasive CT-based radiomics.
Paper Link
|
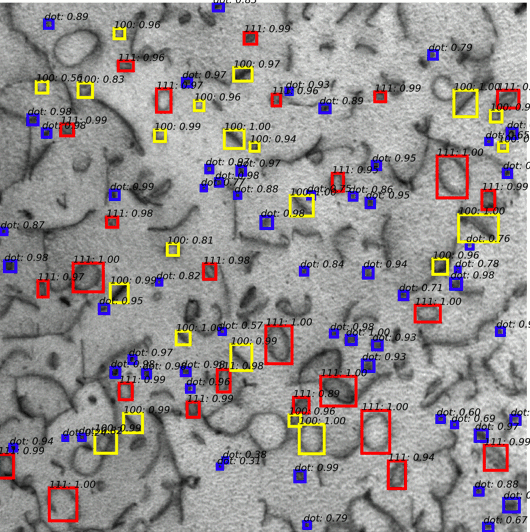 |
Multi defect detection and analysis of electron microscopy images with deep learning
Mingren Shen, Guanzhao Li, Dongxia Wu, Yuhan Liu, Jacob Greaves, Wei Hao, Nathaniel J. Krakauer, Leah Krudy, Jacob Perez, Varun Sreenivasan, Bryan Sanchez, Oigimer Torres, Wei Li, Kevin Field, Dane Morgan, 2021
Electron microscopy is widely used to explore defects in crystal structures, but human detecting of defects is often time-consuming, error-prone, and unreliable, and is not scalable to large numbers of images or real-time analysis. In this work, we discuss the application of machine learning approaches to find the location and geometry of different defect clusters in irradiated steels. We show that a deep learning based Faster R-CNN analysis system has a performance comparable to human analysis with relatively small training data sets. This study proves the promising ability to apply deep learning to assist the development of automated microscopy data analysis even when multiple features are present and paves the way for fast, scalable, and reliable analysis systems for massive amounts of modern electron microscopy data.
Paper Link
|
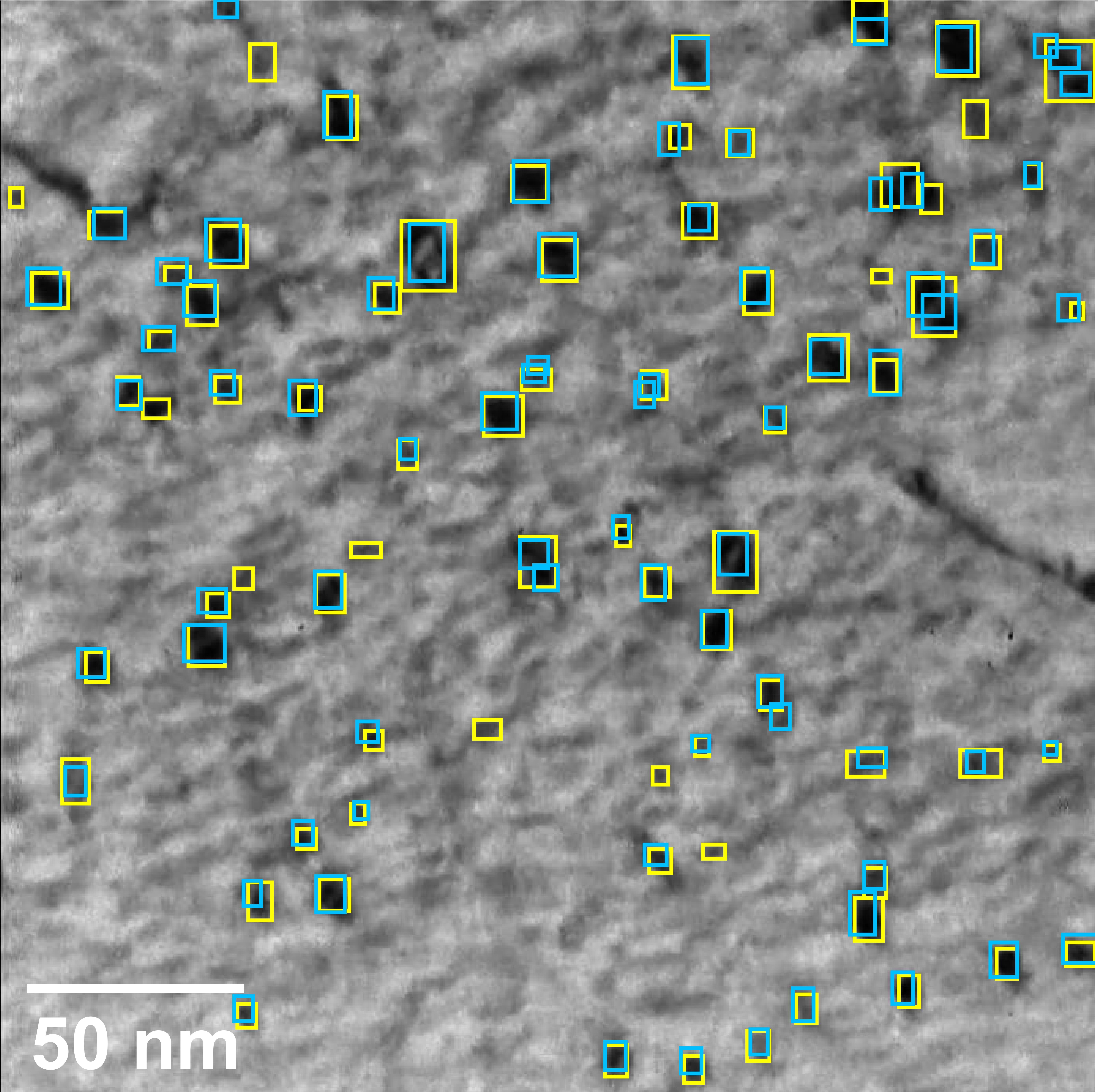 |
A deep learning based automatic defect analysis framework for In-situ TEM ion irradiations
Mingren Shen, Guanzhao Li, Dongxia Wu, Yudai Yaguchi, Jack C.Haley, Kevin G.Field, Dane Morgan, 2021
Videos captured using Transmission Electron Microscopy (TEM) can encode details regarding the morphological and temporal evolution of a material by taking snapshots of the microstructure sequentially. However, manual analysis of such video is tedious, error-prone, unreliable, and prohibitively time-consuming if one wishes to analyze a significant fraction of frames for even videos of modest length. In this work, we developed an automated TEM video analysis system for microstructural features based on the advanced object detection model called YOLO and tested the system on an in-situ ion irradiation TEM video of dislocation loops formed in a FeCrAl alloy. The system provides analysis of features observed in TEM including both static and dynamic properties using the YOLO-based defect detection module coupled to a geometry analysis module and a dynamic tracking module. Results show that the system can achieve human comparable performance with an F1 score of 0.89 for fast, consistent, and scalable frame-level defect analysis. This result is obtained on a real but exceptionally clean and stable data set and more challenging data sets may not achieve this performance. The dynamic tracking also enabled evaluation of individual defect evolution like per defect growth rate at a fidelity never before achieved using common human analysis methods. Our work shows that automatically detecting and tracking interesting microstructures and properties contained in TEM videos is viable and opens new doors for evaluating materials dynamics.
Paper Link
|
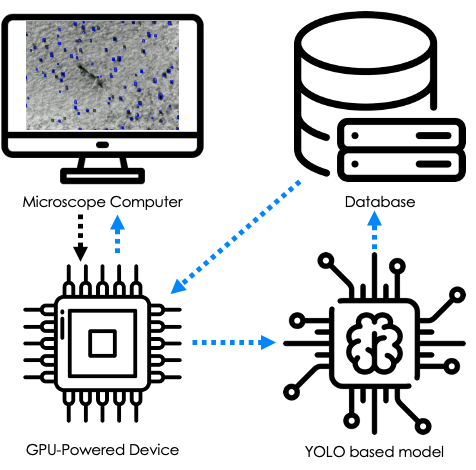 |
Development and Deployment of Automated Machine Learning Detection in Electron Microcopy Experiments
Kevin G. Field, Ryan Jacobs, Mingren Shen, Matthew Lynch, Priyam Patki, Christopher Field, Dane Morgan, 2021
Here, we demonstrate on-going pursuits toward providing automation in electron microscopy images using CNNs. The first pursuit is the application of a lightweight, reduced ordered transmission electron microscopy image simulation method to generate large amounts of "synthetic" data for training common CNN architectures. Specifically, we have developed a Python-based image generation algorithm that uses simplified phase-contrast models to simulate features of interest in either an under- or over-focused bright field Transmission Electron Microscopy (BF-TEM) condition. The output of this physics-based simulation is then integrated with experimentally obtained background images to create novel "synthetic" images with automatically labeled (via both bounding box and pixel segmentation) features.
Paper Link
|
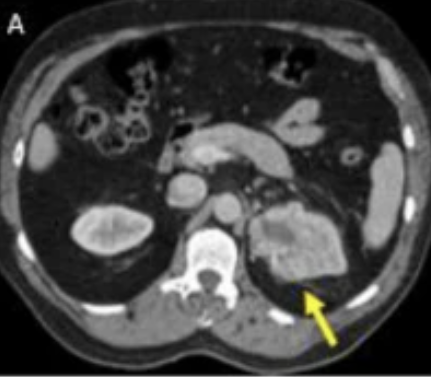 |
Evaluation of radiomics and machine learning in identification of aggressive tumor features in renal cell carcinoma (RCC)
Gurbani, Sidharth, Dane Morgan, Varun Jog, Leo Dreyfuss, Mingren Shen, Arighno Das, E. Jason Abel, and Meghan G. Lubner, 2021
The purpose of this study was to evaluate the use of CT radiomics features and machine learning analysis to identify aggressive tumor features, including high nuclear grade (NG) and sarcomatoid (sarc) features, in large renal cell carcinomas (RCCs).
The XG Boost Model performed best on the tested data. After manual and machine feature extraction, models consisted of 3, 7, 5, 10 radiomics features for NC sarc, PV sarc, NC NG and PV NG, respectively. The area under the receiver operating characteristic curve (AUC) for these models was 0.59, 0.65, 0.69 and 0.58 respectively. The multichannel NG model extracted 6 radiomic features using the feature selection strategy and showed an AUC of 0.67.
Statistically significant but weak associations between aggressive tumor features (high nuclear grade, sarcomatoid features) in large RCC were identified using 3D radiomics and machine learning analysis.
Paper Link
|
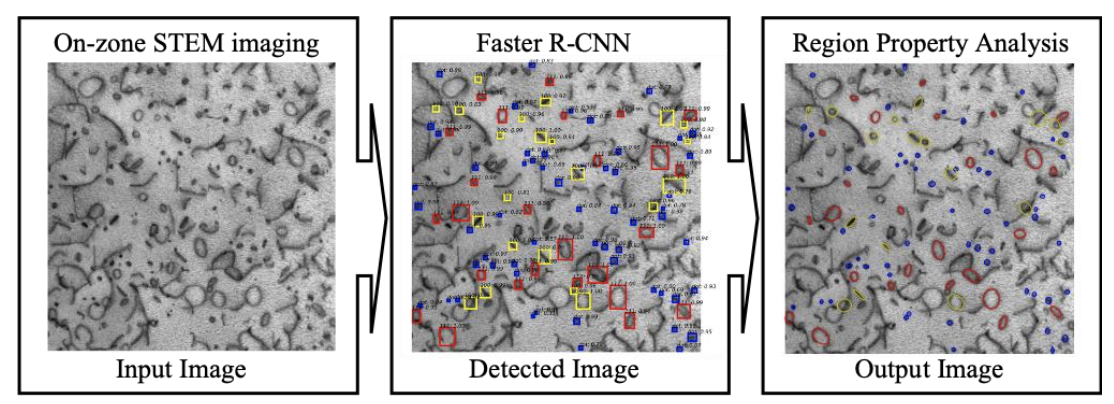 |
Rapid Characterization Methods for Accelerated Innovation for Nuclear Fuel Cladding
Field, Kevin G., Mingren Shen, Caleb P. Massey, Kenneth C. Littrell, and Dane D. Morgan, 2020
Traditional pathways for understanding the performance of nuclear fuel cladding requires irradiations–either via accelerated ion irradiations or through neutron irradiations–followed by some degree of characterization of the microstructure. These characterization efforts have historically included transmission-based electron microscopy techniques and more recently atom probe tomography (APT) techniques. These methods have inherently laborious components within them such as sample preparation and data collection/analysis. These intensive efforts have retarded the speed of innovation for nuclear fuel cladding even with increases in instrument resolution/efficiency.
Here, we demonstrate two on-going pursuits to reduce or completely eliminate the laborious efforts in characterizing irradiation nuclear fuel cladding. The first pursuit is the application of shielded magnetic small angle neutron scattering (SM-SANS) for replacing (or supplementing) APT characterization efforts of nanoscale precipitates in irradiated cladding. The second is the use of a coupled framework for automated dislocation loop detection and analysis based upon on-zone Scanning Transmission Electron Microscopy (STEM) dislocation imaging with deep learning-based feature detection and tracking algorithms. Both pursuits are centralized on the on-going efforts to innovate and maturate the FeCrAl alloy class for Accident Tolerant Fuel (ATF) nuclear fuel cladding [1].
Paper Link
|
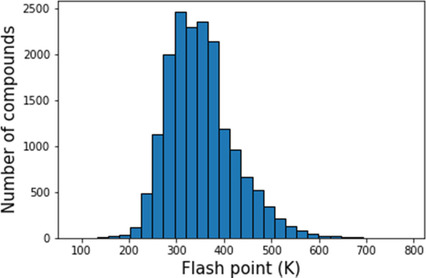 |
Assessing Graph‐based Deep Learning Models for Predicting Flash Point
Xiaoyu Sun, Nathaniel J. Krakauer, Alexander Politowicz, Wei‐Ting Chen, Qiying Li, Zuoyi Li, Xianjia Shao, Alfred Sunaryo, Mingren Shen, James Wang, Dane Morgan, 2020
Flash points of organic molecules play an important role in preventing flammability hazards and large databases of measured values exist, although millions of compounds remain unmeasured. To rapidly extend existing data to new compounds many researchers have used quantitative structure‐property relationship (QSPR) analysis to effectively predict flash points. In recent years graph‐based deep learning (GBDL) has emerged as a powerful alternative method to traditional QSPR. In this paper, GBDL models were implemented in predicting flash point for the first time. We assessed the performance of two GBDL models, message‐passing neural network (MPNN) and graph convolutional neural network (GCNN), by comparing against 12 previous QSPR studies using more traditional methods. Our result shows that MPNN both outperforms GCNN and yields slightly worse but comparable performance with previous QSPR studies. The average R^2 and Mean Absolute Error (MAE) scores of MPNN are, respectively, 2.3 % lower and 2.0 K higher than previous comparable studies. To further explore GBDL models, we collected the largest flash point dataset to date, which contains 10575 unique molecules. The optimized MPNN gives a test data R^2 of 0.803 and MAE of 17.8 K on the complete dataset. We also extracted 5 datasets from our integrated dataset based on molecular types (acids, organometallics, organogermaniums, organosilicons, and organotins) and explore the quality of the model in these classes.
Paper Link
|
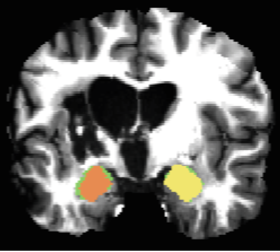 |
Harmonization and Targeted Feature Dropout for Generalized Segmentation: Application to Multi-site Traumatic Brain Injury Images
Yilin Liu, Gregory R. Kirk, Brendon M. Nacewicz, Martin A. Styner, Mingren Shen, Dong Nie, Nagesh Adluru, Benjamin Yeske, Peter A. Ferrazzano, Andrew L. Alexander, 2019
While learning based methods have brought extremely promising results in medical imaging, a major bottleneck is the lack of generalizability. Medical images are often collected from multiple sites and/or protocols for increasing statistical power, while CNN trained on one site typically cannot be well-transferred to others. Further, expert-defined manual labels for medical images are typically rare, making training a dedicated CNN for each site unpractical, so it is important to make best use of the limited labeled source data. To address this problem, we harmonize the target data using adversarial learning, and propose targeted feature dropout (TFD) to enhance the robustness of the model to variations in target images. Specifically, TFD is guided by attention to stochastically remove some of the most discriminative features. Essentially, this technique combines the benefits of attention mechanism and dropout, while it does not increase parameters and computational costs, making it well-suited for small neuroimaging datasets. We evaluated our method on a challenging Traumatic Brain Injury (TBI) dataset collected from 13 sites, using labeled source data of only 14 healthy subjects. Experimental results confirmed the feasibility of using the Cycle-consistent adversarial network for harmonizing multi-site MR images, and demonstrated that TFD further improved the generalization of the vanilla segmentation model on TBI data, reaching comparable accuracy with that of the supervised learning. The code is available at
here
Paper Link
|
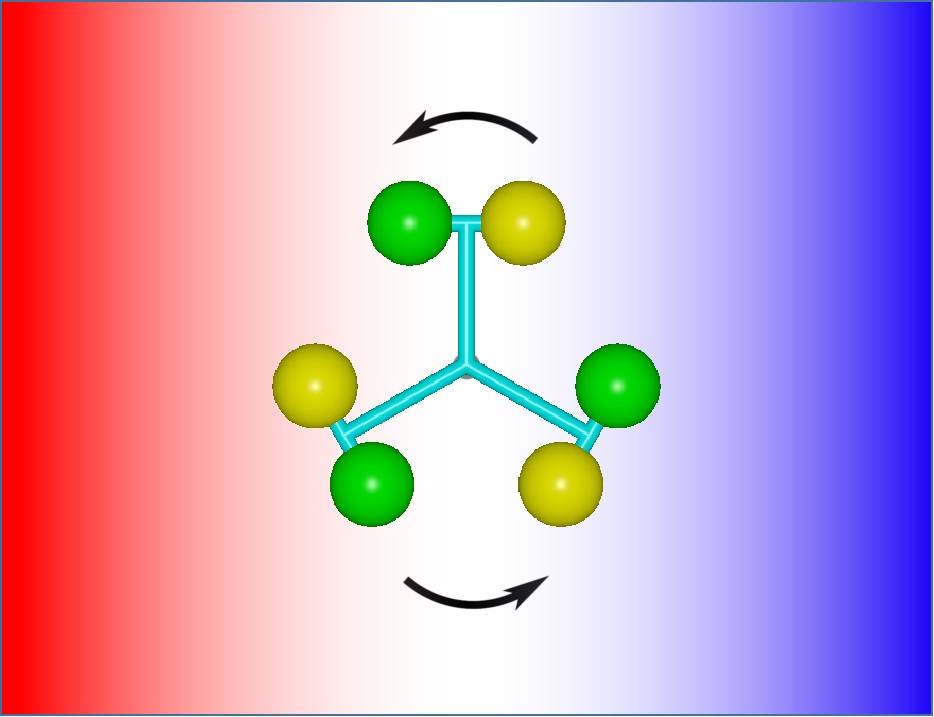 |
Diffusive-Flux-Driven Microturbines by Fore-and-Aft Asymmetric Phoresis
Mingren Shen, Rui Liu, Ke Chen, and Mingcheng Yang, 2019
We designed the microscale turbine that is powered purely by an external diffusive heat or mass flux. The turbine rotates perpendicular to the external thermal or chemical gradient due to fore-and-aft asymmetric thermophoresis or diffusiophoresis. Thus, its performance does not need alignment. The prototype turbine is validated by means of computer simulations. The results show that the angular velocity of the turbine sensitively depends on its structure and interactions with the solvent. This perpendicular-axis phoretic turbine provides large flexibility and the possibility to exploit existing thermal or chemical energies at small scales.
Paper Link
|
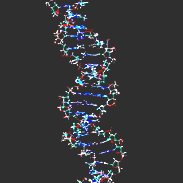 |
N6-methyldeoxyadenosine directs nucleosome positioning in Tetrahymena DNA
Guan-Zheng Luo, Ziyang Hao, Liangzhi Luo, Minren Shen, Daniela Sparvoli, Yuqing Zheng, Zijie Zhang, Xiaocheng Weng, Kai Chen, Qiang Cui, Aaron P. Turkewitz and Chuan He, 2018
I use large scale GPU accelerated molecular dynamics (MD) simulations to study mechanical property changes due to N6-methyldeoxyadenosine of DNA chains. The results shows knockout of a potential 6mA methyltransferase leads to a transcriptome-wide change of gene expression.
Paper Link
|
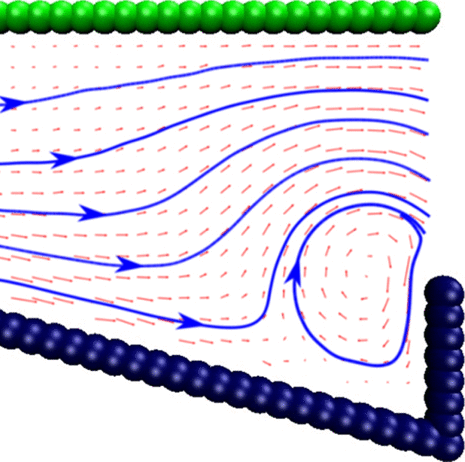 |
Chemically driven fluid transport in long micro channels
Mingren Shen, Fangfu Ye, Rui Liu, Ke Chen, Mingcheng Yang, and Marisol Ripoll, 2016
I show that a concentration drop across micro channels with periodically inhomogeneous boundary walls can laterally transport fluids over arbitrarily long distances along the micro channel. This work thus presents new insight into the fluid transport in long micro channels commonly found in nature and is useful for designing novel micro- or nanofluidic pumps.
Paper Link
|
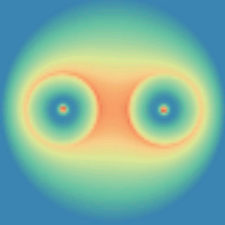 |
Mesoscale simulation of self-diffusiophoretic micro rotor
Mingren Shen, Rui Liu, Mei-Ying Hou , Ming-Cheng Yang , Ke Chen, 2016
I employ hybrid molecular dynamics (MD) simulations and multi-particle collision dynamics (MPC) to investigate the motion of micro rotors. The rotational direction and speed of the micro rotor are determined by bead-solvent interactions, the rotor geometry, the solvent viscosity and the catalytic reaction ratio.
Paper Link(In Chinese)
|
MiNES2021: Discerning the effects of solute additions in FeCrAl on dislocation dynamics under irradiation using a Machine Learning object detection algorithm
American Nuclear Society (ANS), September 19–23, 2021 in Pittsburgh, Pennsylvania, USA
Priyam Patki, Mingren Shen, Yudai Yaguchi, Jack Haley, Dane Morgan, Kevin Field
Poster
|
SAR21: Use of radiomics and machine learning for identification of mucinous pancreas cysts
Society of Abdominal Radiology, 2021, Virtual Meeting
Adam Awe, Michael Vanden Heuvel, Tianyuan Yuan, Mingren Shen, Victoria Rendell (MD), Agrima Kampani, Shanchao Liang, Dane Morgan (PhD), Emily Winslow (MD), and Meghan Lubner (MD)
Oral Presentation
|
ARRS21: Diagnostic potential of machine learning analysis and radiomics for pancreatic cysts
American Roentgen Ray Society, 2021,
Adam Awe, Michael Vanden Heuvel, Tianyuan Yuan, Mingren Shen, Victoria Rendell, Emily Winslow, Meghan Lubner
Oral Presentation
|
MS&T19: Automated Defect Detection in Electron Microscopy with Machine Learning
Materials Science & Technology 2019 - Data Science for Material Property Interpretation (09/29/2019 - 10/03/2019, Portland, OR, USA)
Dane Morgan, Mingren Shen, Wei Li, Kevin Field
Oral Report
|
CIRSE 2019: Identifying active extravasation on arteriograms using artificial intelligence
Cardiovascular and Interventional Radiological Society of Europe (CIRSE) (09/07/2019 - 09/11/2019, Barcelona, Spain)
Mingren Shen, Mesut Ozturk, Po-Ling Loh, Varun Jog, Paul Laeseke, Dane Morgan, Mark Kleedehn
Oral Report: 2306.7 - Identifying active extravasation on arteriograms using artificial intelligence
|
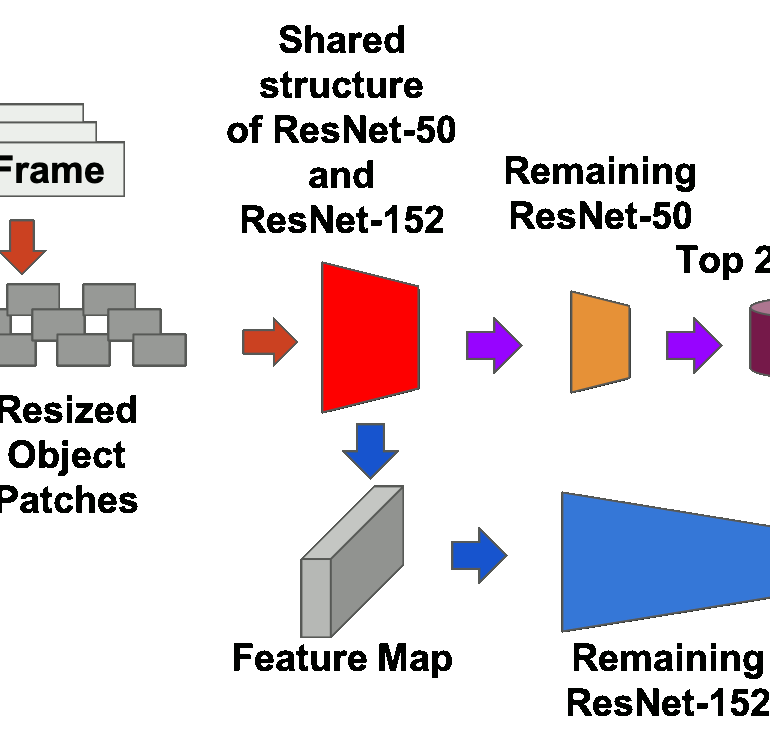 |
Query time optimized video inference system
Mingren Shen, Shuoxuan Dong, Xiuyuan He, 2018
Optimizing the latency of a two CNNs video inference system by reusing the intermediate results of first CNN(ResNet50 ) to accelerate the calculation of second CNN( ResNet152 ). We successfully achieved 18% latency decrease without sacrificing the accuracy of the model.
Final Report
|
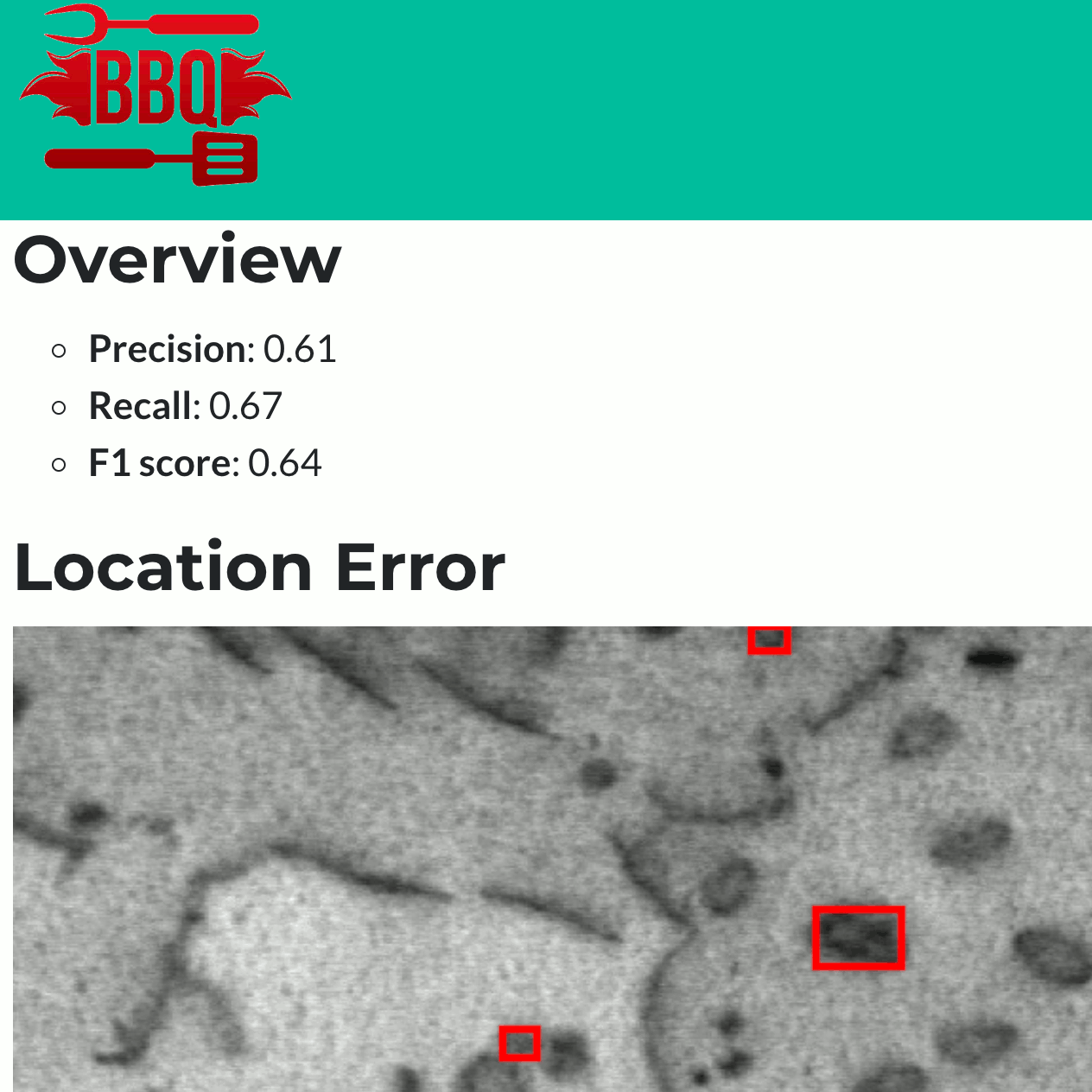 |
BBQ:Bounding Box Quality Checker
Mingren Shen, Xiuyuan He, 2018
BBQ standing bounding box qualifier which is a web service that examines the matching between ground truth bounding boxes and the predicted bounding boxes and produces a prediction quality report and debugging tools for object detection algorithm. A lot of work of object detection methods are focusing on how to generate bounding box annotations. However, few have talked about how to debug or check where the object detection model fails. This Hackathon project is a tool that can help people actually see where the object detection algorithm fails.
Github
|
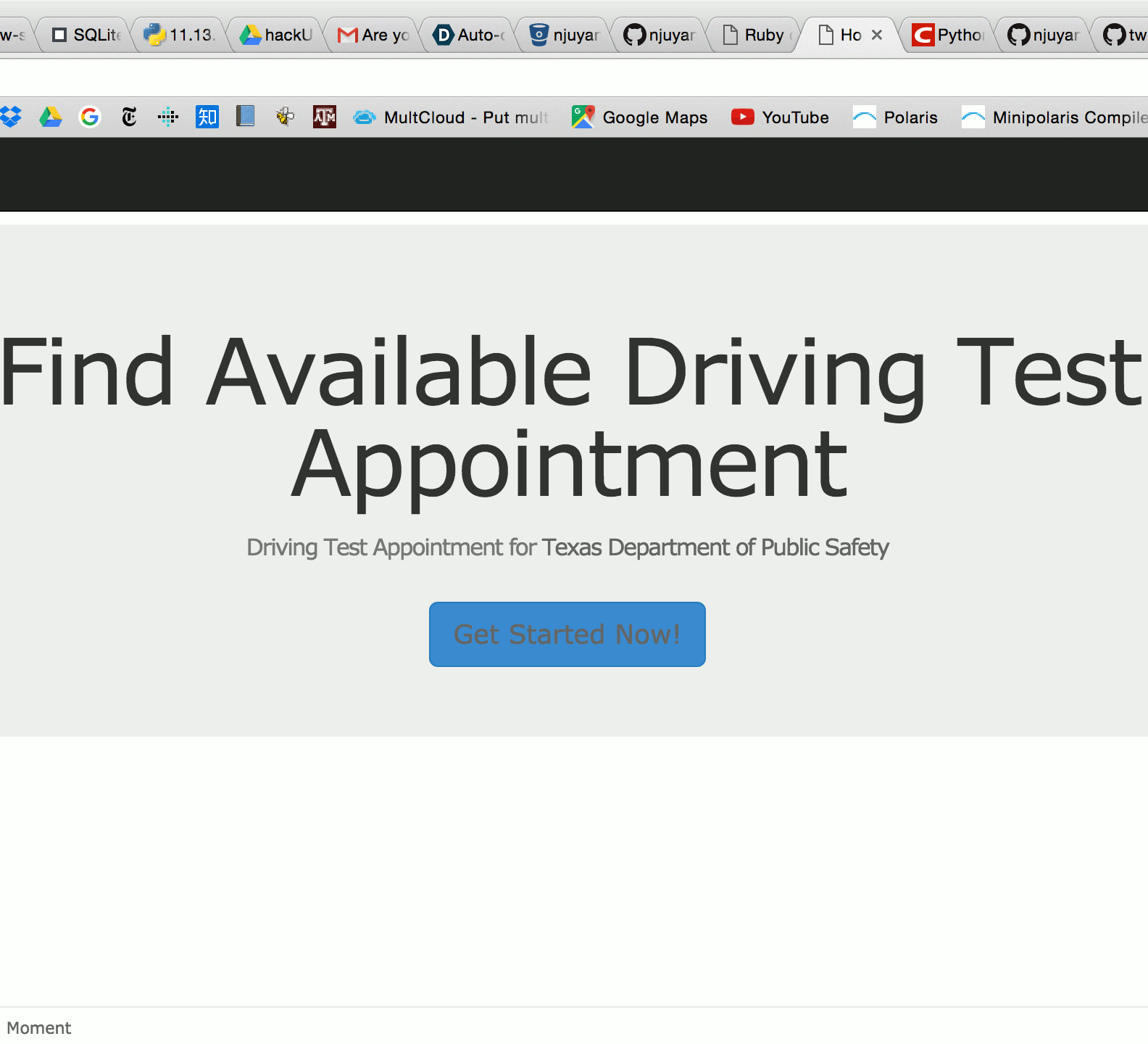 |
Driver Test Schedule System
Mingren Shen , Yang Yang, Jiachen Shen, 2016
This project helps reminder the users when there are personalized available space for their driving test in College Town of TAMU. The project is build on Ruby on Rails,Ruby(4.2.2),Python.
Github
|
|